Introduction to linear regression:
Linear regression is the first prediction algorithm taught in statistics to the students who begin their journey in the line of prediction and prescribing and doing all sorts of statistics wizardry. Being a statistics student, in this post, I will provide a introduction to linear regression for people not so into maths and go into bit by bit into the details, use of linear regression in different statistical packages and therefore try to balance it out for people looking at linear regression from statistics point of view by helping them into getting the application side.
In this post, we will discuss about the following topics:
- what is linear regression
- different types of linear regression
- What are the applications of linear regressions?
- How to interpret results of linear regressions?
- adjusted R-square and mean-squared-error
- coefficients and their significance
- Assumptions of linear regression
- assumption of multivariate-normality
- what is homoskedascity
- assumption of independence of the predictor variables
- assumption of no autocorrelation
- analytical and theoretical solution of a linear regression
- verification of assumptions in linear regression
- how to check multicollinearity
- How to implement linear regression
What is linear regression?
In general, a perfectly linear relation is generally hard to expect. But surprisingly enough, a linear model, being basic enough though, behaves pretty well to predict and explain the effects of different independent variables on a target/dependent variable.
different types of linear regression:
What are the applications of linear regressions?
How to interpret results of linear regressions?
(1) adjusted R-square and mean-squared-error:
(2) coefficients and their significance:
Once you understand the statistical background in details, you will be able to interpret it in several other ways. Now, let's head into the first steps of solving a linear regression problem.
Assumptions of linear regression:
The equation of multivariate linear regression is:
βε
where β is a vector of co-efficients of the variables, X is the design matrix and ε is a vector of errors.
Now, let us dive into the assumptions:
(1) assumption of multivariate-normality:
or in case of multivariate linear regression, it is assumed that,
ε ~ N(0, Σ)
Where:
- ε (epsilon) represents the error term.
- Σ (uppercase sigma) represents the correlation matrix of the errors.
- Ļ (lowercase sigma) represents the standard deviation.
This notation indicates that the error term ε follows a normal distribution N with a mean of 0 and a covariance matrix Σ.
(2) homoskedascity:
(3) independence of the predictor variables:
(4) Linear relationship:
(5) No autocorrelation:
Analytical solution of Linear regression:
Solving the linear regression is quite a bit technical. Let's start with the problem equation again and what we want to solve. In the equation,Hereās the transformed and rewritten version of your expression with symbols:
Y = βX + ε
Where:
- β (beta) are the unknown parameters we want to predict.
- ε (epsilon) is the error term.
To estimate the unknown parameter β, we want to minimize the norm of the errors. We aim to minimize the expression:
ā ( Y - βX )įµ ( Y - βX ) ā
To find the value of β that minimizes the norm, we take the derivative of the expression inside the norm with respect to β and set it equal to 0. After performing the derivation, we obtain:
β = (XįµX)ā»Ā¹ XįµY
Where:
- Xįµ is the transpose of matrix X.
- (XįµX)ā»Ā¹ is the inverse of the matrix XįµX.
- Y is the observed data.
This is the ordinary least squares (OLS) solution for estimating β.
So, there it is, the theoretical solution of linear regression. Now, here are some technicalities which I am not going to further delve into. You can derive the standard deviations of β, i.e. the coefficients and then formulate the standard t-test for significance of the co-efficients. For further details, please follow this awesome stat-book.How to use Linear regression
There are two parts of a linear regression use in practical cases. First one is the verification of the assumptions and the second one is the fitting of the dataset in a linear regression packages. We will first talk about how to do the checking of the assumptions.verification of assumptions
assumptions of normality:
To check normality of the errors, one can run the linear regression, calculate the errors, and then check the errors for normality. For normality check, there are multiple tests, but you can use shapiro-wilk normality test.assumptions of linear relationship:
To check linear relationship, you have to plot the target variable vs the predictor variable. The plot, if surrounds around a line, then it supports a linear relationship, while if it is scattered circularly, then it doesn't support linear relationship. This idea is also depicted by the pearson correlation of the predictor variable and target variables. In general, if the correlation is below , then there is no or little relation between the predictor and the target variable. One other way to check if there is any correlation is to create a random data sample, with a similar range to that of the predictor variable and then check its correlation and plots with the predictor variable. If it is similar to that of the target variable vs predictor variable then clearly the predictor variable doesn't have any significant variable and if the predictor variable has a significantly better correlation and linear plot with target variable then, it means there is some amount of correlation and linear relationship with between target and predictor variable.multi-collinearity check and prevention:
what is multi-collinearity?
Often in a dataset, predictor variables are dependent and highly correlated with each other. This is called multi-collinearity. Often this is a doubt that how high the correlation should be to call two columns as highly collinear and it needs attention. For this, generally, you can consider 0.9 as a correlation, but if you are too restrictive then you can even consider correlations higher than 0.75 as highly correlated. More discussion on this can be followed here on stackoverflow.how can we check multicollinearity?
There are quite several ways to check multicollinearity.The first and most popular one way is to find out VIF. VIF is the variance inflation factor.VIF of a predictor variable is a measure of R-squared for the regression of the predictor variable being predicted by all the other predictor variables. So if VIF is high, it means that the corresponding variable can be predicted well by the other variables, therefore high multicollinearity is present. For a general rule of thumb, if VIF is greater than 10, definitely multi-collinearity is present, while a VIF 5-10 also suggests some amount of multicollinearity. One other similar term you may have heard about, which is tolerance. Tolerance is the inverse of vif, and just from what we discussed above, a tolerance less than 0.1 denotes high multi-collinearity.
There are certainly other ways to find multi-collinearity. To start with the procedures, one can start checking correlations between pairs of the predictor variables and then if you find high correlations, then that is a sign of multicollinearity.
Also, you can check standard deviations of the predictor variables. The high variance of the predictor variables often denotes that some of the predictor variables have high collinearity and therefore the estimates are not accurate enough to have low variances.
Other than that, you can consider business points of view for the coefficients. Often from the business or context of the linear regression, you will have an idea about which variables should have high coefficients of variables and which variables should influence the target variable in which direction. But generally, the coefficients will not have the proper sizes, or will not show the proper signs in general when collinearity shows up. These are concepts that are not direct ways to analyze but ways to realize or detect multicollinearity once you have run your regression already.
There are other ways to find out multicollinearity, as such condition index, but these are way less obvious and direct than the ways I have mentioned already.
How to solve non-normality?
The other problems, i.e. non-normality and homoskedasticity can also be solved sometimes using probabilistic methods. Beta distributions, chi-square distributions, and many other distributions can be transformed into normal distributions using specific transformations. Therefore, whenever errors do not satisfy the normality, one can transform the error vector in a way to fit it into normality and therefore apply the linear transformation solution.
How to solve non-linear relationship problem between predictor and target variable?
Also, when there is evidence of a lack of linear relationship between a predictor variable and a target variable, but they are correlated, then one can look at the corresponding graph.These graphs let us know that often the target variables are polynomially or logarithmically connected with the predictor variable. In these cases, we can use the polynomials or log of that predictor variable as a new variable instead of that predictor variable as that transformation is in linear relation with the target variable. This is the way one remedies the problem of not having a linear relationship between the predictor and the target variable. If even after transformation there is not much correlation, then one should not use that predictor variable anyway.
How to implement linear regression:
There is a number of statistical languages to use linear regression on. We will discuss how to use linear regression on R, Python, Julia, and several other languages. Please read through this section as this is the most applied section as you can use similar codes whenever you need to use linear regression in practical cases.
Linear regression using python:There are two packages in python to use linear regression. One of them is statsmodels.api where the other one is sklearn.linear_model. First I will discuss what is the difference between these two and when you should use which one. The statsmodels package is one that gives the statistical significance of the features used in regression and therefore, statsmodels are important when you run a linear regression for sake of feature importance analysis, t-test of features and verify other statistical significances. But as it not only solves the linear regression parameter optimization but also runs all these tests, checks significances and other things, therefore, this package is significantly slower than the sklearn package when you run the regression on a moderate amount of data, i.e. million rows and so on. Therefore, when you want more of a prediction sort of work to be done by the regression, and don't want to focus on the statistical tests or significances, then sklearn is clearly a better choice to go with, as it will be fast and easier to implement.
Also other than clarifying this point, I will want to clarify one more difference between sklearn and statsmodels. sklearn fits the linear regression by default with an intercept variable i.e. a column of 1 is taken as a predictor variable which is analogous to the intercept in case of a simple 2-dimensional linear regression. But by default, the statsmodel doesn't fit an intercept variable. That's why, whenever you want to take an intercept variable which you should always; if not specified otherwise by some specific theory, then you have to manually add that column of 1 to your data and then you should pass your data to the statsmodels.api.
sklearn linear regression:
Now, look at how we apply the sklearn linear regression on a dataset available here.We will use the datasets from the sklearn. You can access datasets available from sklearn, using sklearn.datasets. We will take the load_boston command to load the Boston data. I will not go details about what and how the data is collected, but if you want to explore the Boston data on your own, please follow this link to know more about the data. Now in the following blocks of codes, see how the linear regression from sklearn is implemented.

This next screenshot shows that how to import linear regression using LinearRegression from sklearn.linear_model, how to fit a model using existing data and also how to predict for new data using the predict attribute.
I have also imported and included the use of the general accuracy check and feature importance check. For all sorts of accuracy metrics, one generally has to use sklearn.metrics library. In this specific case, as I discussed mean_squared_error to be already a good check for the accuracy or error rate of linear regression, I have imported mean_squared_error from sklearn.metrics and used it to check the accuracy of the linear regression. Mainly the values to be predicted are in 20-30 range, and we have got a mse of 21.84, which is around an error of 4.5-4.6 on the average; which is a mediocre level of prediction and a type of accuracy you can expect from linear regression on normal data. Also, we have imported the R squared score as it is one of the other significant markers of the accuracy of linear regression.
For the R-square score, one uses the R2-score function from the sklearn.metrics module. It is also as easy to use as the mean squared error.
One other noticeable point from programming nicety view is that how I have imported these functions with big names as small abbreviations. This eases up both writing time and usage over the code. Although this doesn't have any efficiency effect on the programming just to be clear.
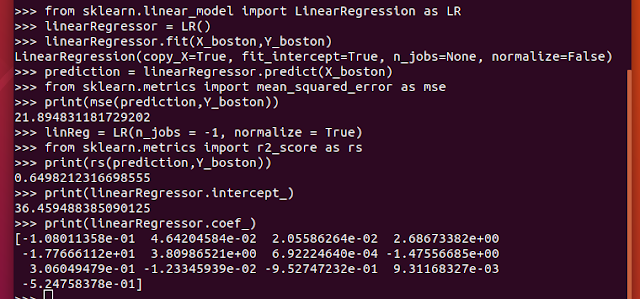
We have achieved a 64% Rsquare in the linear regression made in the following block; which is kind of a good explainability. There will always be project-specific requirement in your modeling though which will sometime become more important than just mse and r-square, but those are generally beyond statistical scope of linear regression and therefore no need to discuss in this blog.
Next lets see how we can use statsmodel package on the same data. We will just show the package use part as we already have the data loaded.
Comments
Post a Comment